Revolutionizing Plywood Quality: Grading and defect detection with AI

A proven player in industrial automation, MJV Automation boasts unparalleled efficiency and reliability. While stationed in Finland, their reach extends globally, with major part of their revenue from international projects. www.mjvautomation.fi
Automation
10+ employees
“The collaboration with Lateral Engine enabled us to provide solutions and quality that would have otherwise been out of our reach in the fields of Neural networks and image processing. This boosted our journey towards offering new technologies and products to our customers.”
Jussi Rautiainen, Managing Director
Challenge
The mechanical forestry industry is synonymous with precision and quality. Plywood, a core product, must meet rigorous standards to ensure strength, durability, and aesthetics. Historically, pinpointing defects, especially intricate details like branches within the plywood sheets, proved to be a daunting task. Traditional methods of quality assurance were primarily manual, often requiring highly trained professionals to inspect sheets as they moved by on a production line. Such methods were not only time-consuming but were also prone to human error, resulting in wasted material and compromised product quality.
Solution
Understanding the criticality of the challenge at hand, our team set out to harness the unparalleled capabilities of Deep Neural Networks (DNN) to overhaul the quality assurance process. Our solution comprised:
State-of-the-art Image Processing: As sheets of plywood move through the production line, our advanced imaging systems capture high-resolution images, ensuring every detail, no matter how minute, is captured.
Deep Neural Network Integration: These captured images are fed into a DNN, which has been meticulously trained to recognize and flag even the tiniest of defects. Our emphasis on targeting branches within the plywood allowed the system to understand the nuanced differences between natural wood patterns and actual imperfections.
Comprehensive User Interface: Tailored to the specific needs of operators, our interface consists of:
- Dashboard: A runtime dashboard provides operators with a real-time view of the system’s grading and throughput. This intuitive dashboard is customizable, allowing operators to modify and prioritize the data they wish to see.
- Databrowser: Serving as a repository of results, the databrowser allows operators to filter and review defect specifics of plywood sheets. Furthermore, it integrates a grading matrix, visualizing the grading logic. This matrix clearly highlights identified issues, enabling operators to discern defect categories like “rot” area, “hole” occurrences, and more.
User-Friendly Training Tool: This easy-to-use feature allows operators to label new data, ensuring the DNN remains up-to-date with evolving quality parameters and standards. This iterative learning guarantees the software’s sustained efficiency and accuracy.
Prototyping the lightning and imaging solution
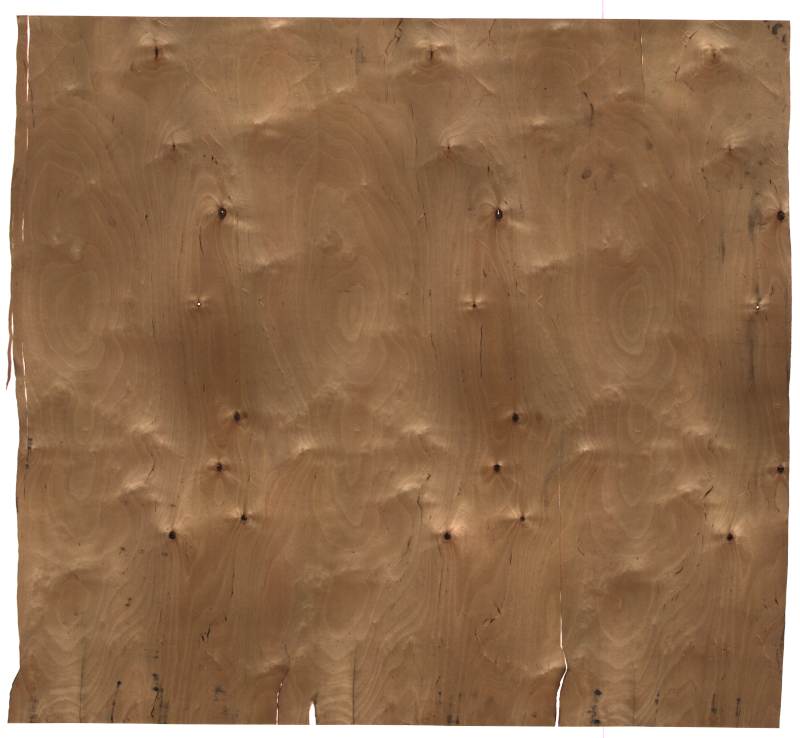
One of the images used in AI training
Result
Reduced Manual Labor: The AI-driven system considerably reduced the need for manual inspections, freeing up skilled professionals for other critical tasks.
Enhanced Detection Rate: Our DNN-driven approach significantly improved defect detection, ensuring that even the minute branches and other imperfections didn’t go unnoticed.
Reduced Wastage: By pinpointing defects early in the production process, manufacturers could rectify issues or repurpose material, leading to a significant reduction in waste.
Empowered Users: The inclusion of the DNN training tool equipped users with the autonomy to further refine the system, ensuring its continued adaptability and relevance.
In partnership with our industrial automation client, we have not only redefined quality assurance in the mechanical forestry sector but also showcased the boundless potential of DNN in revolutionizing industry standards.